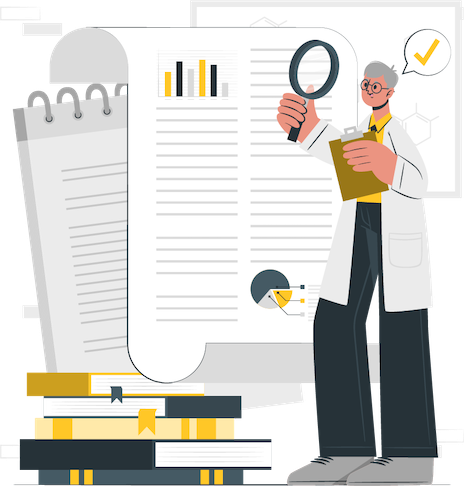
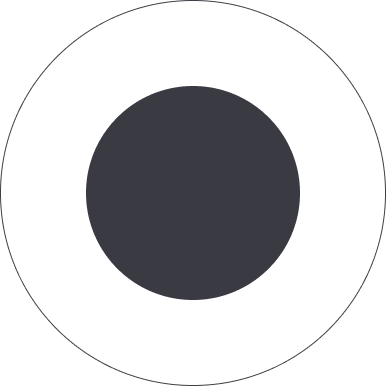
Reasearch Work
My area of interest are AI, Machine learning,
computer vision, and Natural Language Processing.
computer vision, and Natural Language Processing.


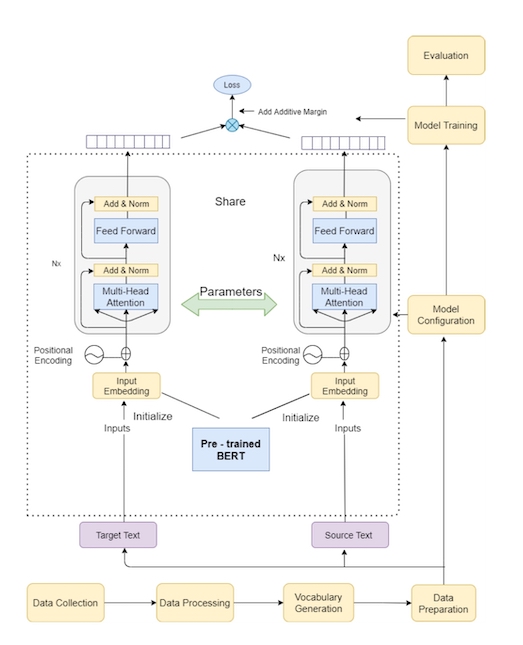
Bangla-BERT: Transformer-based Efficient Model for Transfer Learning and Language
Understanding IEEE Access ·
IEEE Access, 2022
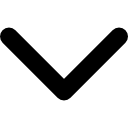
This paper introduces Bangla-BERT, a monolingual BERT model for the Bangla language. Despite the limited data available for NLP tasks in Bangla, we perform pre-training on the largest Bangla language model dataset, BanglaLM, which we constructed using 40 GB of text data. Bangla-BERT achieves the highest results in all datasets and vastly improves the state-of-the-art performance in binary linguistic classification, multilabel extraction, and named entity recognition, outperforming multilingual BERT and other previous research.This paper introduces Bangla-BERT, a monolingual BERT model for the Bangla language. Despite the limited data available for NLP tasks in Bangla, we perform pre-training on the largest Bangla language model dataset, BanglaLM, which we constructed using 40 GB of text data. Bangla-BERT achieves the highest results in all datasets and vastly improves the state-of-the-art performance in binary linguistic classification, multilabel extraction, and named entity recognition, outperforming multilingual BERT and other previous research.
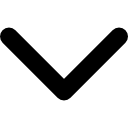
Kowsher, M., Sami, A. A., Prottasha, N. J., Arefin, M. S., Dhar, P. K., & Koshiba, T. (2022). Bangla-BERT: transformer-based efficient model for transfer learning and language understanding. IEEE Access, 10, 91855-91870.
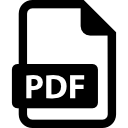
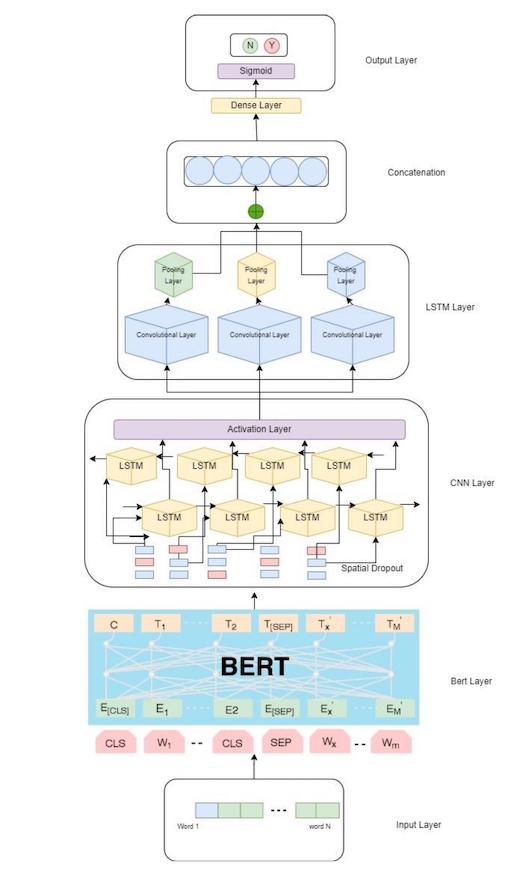
Transfer Learning for Sentiment Analysis Using BERT Based Supervised Fine-Tuning·
Sensors , 2022
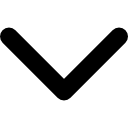
The growth of the Internet has expanded the amount of data expressed by users across multiple platforms. The availability of these different worldviews and individuals’ emotions empowers sentiment analysis. However, sentiment analysis becomes even more challenging due to a scarcity of standardized labeled data in the Bangla NLP domain. The majority of the existing Bangla research has relied on models of deep learning that significantly focus on context-independent word embeddings, such as Word2Vec, GloVe, and fastText, in which each word has a fixed representation irrespective of its context. Meanwhile, context-based pre-trained language models such as BERT have recently revolutionized the state of natural language processing. In this work, we utilized BERT’s transfer learning ability to a deep integrated model CNN-BiLSTM for enhanced performance of decision-making in sentiment analysis. In addition, we also introduced the ability of transfer learning to classical machine learning algorithms for the performance comparison of CNN-BiLSTM. Additionally, we explore various word embedding techniques, such as Word2Vec, GloVe, and fastText, and compare their performance to the BERT transfer learning strategy. As a result, we have shown a state-of-the-art binary classification performance for Bangla sentiment analysis that significantly outperforms all embedding and algorithms
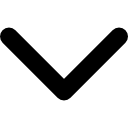
Cite: Prottasha, N. J., Sami, A. A., Kowsher, M., Murad, S. A., Bairagi, A. K., Masud, M., & Baz, M. (2022). Transfer learning for sentiment analysis using BERT based supervised fine-tuning. Sensors, 22(11), 4157.
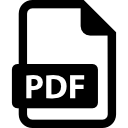
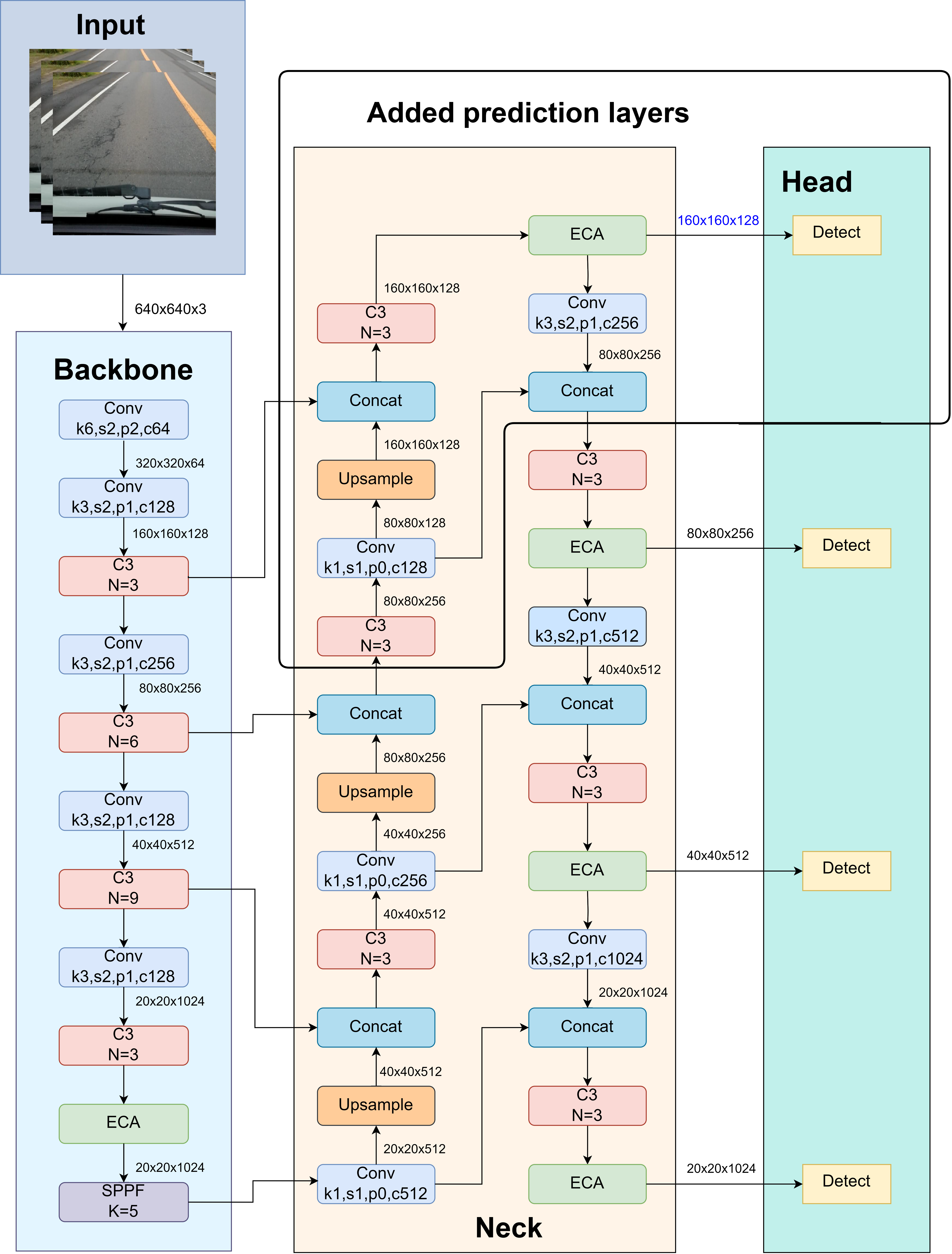
Improved YOLOv5-Based Real-Time Road Pavement Damage Detection in Road Infrastructure Management
Algorithms, 2023
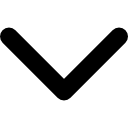
Deep learning has enabled a straightforward, convenient method of road pavement infrastructure management that facilitates a secure, cost-effective, and efficient transportation network. Manual road pavement inspection is time-consuming and dangerous, making timely road repair difficult. This research showcases You Only Look Once version 5 (YOLOv5), the most commonly employed object detection model trained on the latest benchmark Road Damage Dataset, Road Damage Detection 2022 (RDD 2022). The RDD 2022 dataset includes four common types of road pavement damage, namely vertical cracks, horizontal cracks, alligator cracks, and potholes. This paper presents an improved deep neural network model based on YOLOv5 for real-time road pavement damage detection in photographic representations of outdoor road surfaces, making it an indispensable tool for efficient, real-time, and cost-effective road infrastructure management. The YOLOv5 model has been modified to incorporate several techniques that improve its accuracy and generalization performance. These techniques include the Efficient Channel Attention module (ECA-Net), label smoothing, the K-means++ algorithm, Focal Loss, and an additional prediction layer. In addition, a 1.9% improvement in mean average precision (mAP) and a 1.29% increase in F1-Score were attained by the model in comparison to YOLOv5s, with an increment of 1.1 million parameters. Moreover, a 0.11% improvement in mAP and 0.05% improvement in F1 score was achieved by the proposed model compared to YOLOv8s while having 3 million fewer parameters and 12 gigabytes fewer Giga Floating Point Operation per Second (GFlops) .
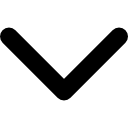
Sami, A. A., Sakib, S., Deb, K., & Sarker, I. H. (2023). Improved YOLOv5-Based Real-Time Road Pavement Damage Detection in Road Infrastructure Management. Algorithms, 16(9), 452.
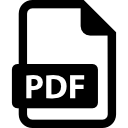
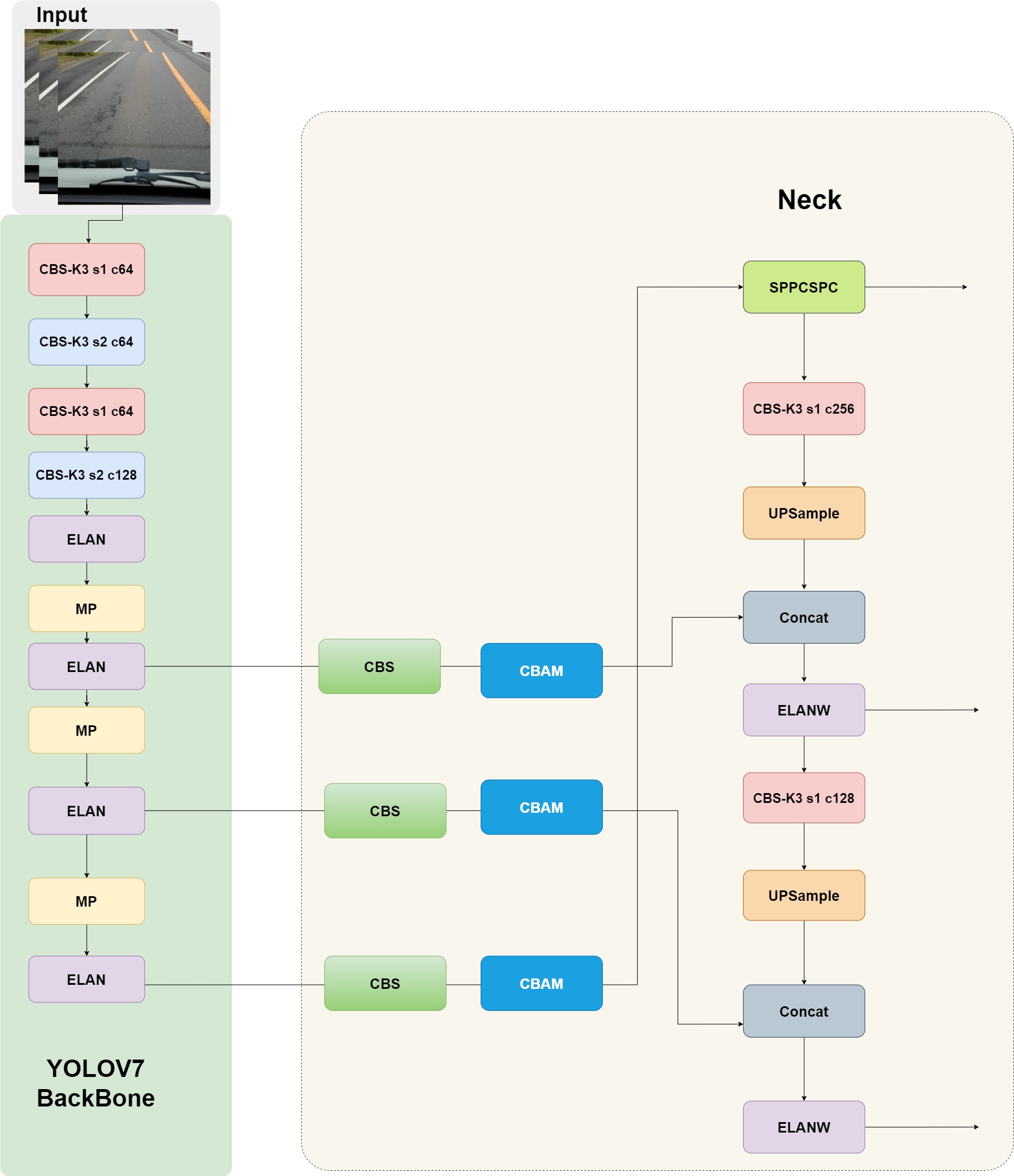
Road Damage Detection and Classification using YOLOv7
Secured Acceptance for a Book Chapter in Taylor & Francis
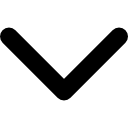
Deep learning has allowed a simple, convenient method of road infrastructure management to facilitate a safe, cost-effective, and efficient transportation network. Recent advancements in deep learning allow the automatic collection and assessment of road damage data. This paper describes the YOLOv7 object detection model trained on the most recent benchmark Road Damage Dataset, RDD 2022. Few studies examined the efficacy of the new state-of-the-art RDD 2022, which comprised six country’s road damage, including Japan, Norway, The USA, Czech, and China, with four common categories of damage, i.e., verticle cracks, horizontal cracks, alligator cracks, and pathhole. We examined different object detection models and concluded that the object detection model YOLOv7 could be the benchmark model for road damage detection and classification. Firstly, we added the Convolutional Block Attention Module (CBAM) module to the network. Secondly, the K-means++ algorithm was employed to determine a suitable anchor box for our dataset. The proposed YOLOv7 exceeded all previous object detection approaches on road damage detection and classification tasks, obtaining 68.61% mAP and a 66.87% F1 score.
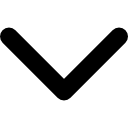
Has been accepted for presentation at the International Conference on Big Data, IoT, and Machine Learning 2023 (BIM 2023), set to be published by Taylor & Francis.
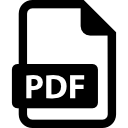
Complete researchs
These all are research profile.
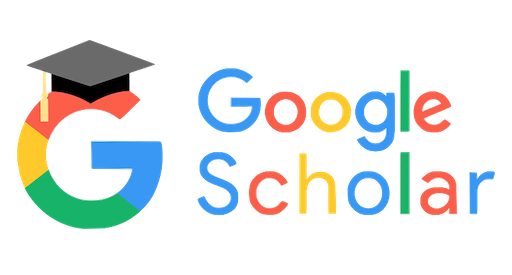
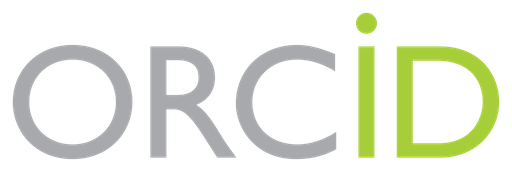
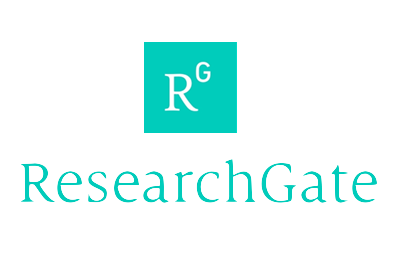